Common Loss functions in machine learning-
1.Mean Square Error/Quadratic Loss/L2 Loss
Mathematical formulation:

import numpy as npy_hat = np.array([0.000, 0.166, 0.333])
y_true = np.array([0.000, 0.254, 0.998])def rmse(predictions, targets):
differences = predictions - targets
differences_squared = differences ** 2
mean_of_differences_squared = differences_squared.mean()
rmse_val = np.sqrt(mean_of_differences_squared)
return rmse_valprint("d is: " + str(["%.8f" % elem for elem in y_hat]))
print("p is: " + str(["%.8f" % elem for elem in y_true]))rmse_val = rmse(y_hat, y_true)
print("rms error is: " + str(rmse_val))
2.Mean Absolute Error/L1 Loss
Mathematical formulation:-

Algorithm-
import numpy as npy_hat = np.array([0.000, 0.166, 0.333])
y_true = np.array([0.000, 0.254, 0.998])
print("d is: " + str(["%.8f" % elem for elem in y_hat]))
print("p is: " + str(["%.8f" % elem for elem in y_true]))
def mae(predictions, targets):
differences = predictions - targets
absolute_differences = np.absolute(differences)
mean_absolute_differences = absolute_differences.mean()
return mean_absolute_differencesmae_val = mae(y_hat, y_true)
print ("mae error is: " + str(mae_val))
3.Mean Bias Error
Mathematical formulation:-
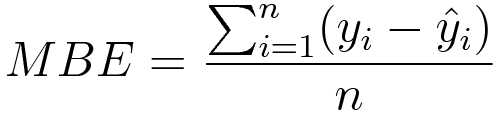
This is much less common in the machine learning domain as compared to its counterpart. This is the same as MSE with the only difference that we don’t take absolute values. Clearly, there’s a need for caution as positive and negative errors could cancel each other out. Although less accurate in practice, it could determine if the model has a positive bias or negative bias.
In simple terms, the score of correct category should be greater than sum of scores of all incorrect categories by some safety margin (usually one). And hence hinge loss is used for maximum-margin classification, most notably for support vector machines. Although not differentiable, it’s a convex function which makes it easy to work with usual convex optimizers used in machine learning domain.
Mathematical formulation :-

Consider an example where we have three training examples and three classes to predict — Dog, cat and horse. Below the values predicted by our algorithm for each of the classes :-

Computing hinge losses for all 3 training examples :-
## 1st training example
max(0, (1.49) - (-0.39) + 1) + max(0, (4.21) - (-0.39) + 1)
max(0, 2.88) + max(0, 5.6)
2.88 + 5.6
8.48 (High loss as very wrong prediction)## 2nd training example
max(0, (-4.61) - (3.28)+ 1) + max(0, (1.46) - (3.28)+ 1)
max(0, -6.89) + max(0, -0.82)
0 + 0
0 (Zero loss as correct prediction)## 3rd training example
max(0, (1.03) - (-2.27)+ 1) + max(0, (-2.37) - (-2.27)+ 1)
max(0, 4.3) + max(0, 0.9)
4.3 + 0.9
5.2 (High loss as very wrong prediction)
2.Cross-Entropy Loss/Negative Log-Likelihood
This is the most common setting for classification problems. Cross-entropy loss increases as the predicted probability diverge from the actual label.
Mathematical formulation:-

Notice that when actual label is 1 (y(i) = 1), second half of function disappears whereas in case actual label is 0 (y(i) = 0) first half is dropped off. In short, we are just multiplying the log of the actual predicted probability for the ground truth class. An important aspect of this is that cross entropy loss penalizes heavily the predictions that are confident but wrong.
import numpy as nppredictions = np.array([[0.25,0.25,0.25,0.25],
[0.01,0.01,0.01,0.96]])
targets = np.array([[0,0,0,1],
[0,0,0,1]])def cross_entropy(predictions, targets, epsilon=1e-10):
predictions = np.clip(predictions, epsilon, 1. - epsilon)
N = predictions.shape[0]
ce_loss = -np.sum(np.sum(targets * np.log(predictions + 1e-5)))/N
return ce_losscross_entropy_loss = cross_entropy(predictions, targets)
print ("Cross entropy loss is: " + str(cross_entropy_loss))
Introduction to Loss Functions
The loss function is the bread and butter of modern machine learning; it takes your algorithm from theoretical to practical and transforms neural networks from glorified matrix multiplication into deep learning.
This post will explain the role of loss functions and how they work, while surveying a few of the most popular from the past decade.
What’s a Loss Function?
At its core, a loss function is incredibly simple: it’s a method of evaluating how well your algorithm models your dataset. If your predictions are totally off, your loss function will output a higher number. If they’re pretty good, it’ll output a lower number. As you change pieces of your algorithm to try and improve your model, your loss function will tell you if you’re getting anywhere.
In fact, we can design our own (very) basic loss function to further explain how it works. For each prediction that we make, our loss function will simply measure the absolute difference between our prediction and the actual value. In mathematical notation, it might look something like abs(y_predicted – y). Here’s what some situations might look like if we were trying to predict how expensive the rent is in some NYC apartments:
Notice how in the loss function we defined, it doesn’t matter if our predictions were too high or too low. All that matters is how incorrect we were, directionally agnostic. This is not a feature of all loss functions: in fact, your loss function will vary significantly based on the domain and unique context of the problem that you’re applying machine learning to. In your project, it may be much worse to guess too high than to guess too low, and the loss function you select must reflect that.
Different Types and Flavors of Loss Functions
A lot of the loss functions that you see implemented in machine learning can get complex and confusing. Consider this paper from late 2017, entitled A Semantic Loss Function for Deep Learning with Symbolic Knowledge. There’s more in that title that I don’t understand than I do. But if you remember the end goal of all loss functions–measuring how well your algorithm is doing on your dataset–you can keep that complexity in check.
We’ll run through a few of the most popular loss functions currently being used, from simple to more complex.
Mean Squared Error
Mean Squared Error (MSE) is the workhorse of basic loss functions: it’s easy to understand and implement and generally works pretty well. To calculate MSE, you take the difference between your predictions and the ground truth, square it, and average it out across the whole dataset.
Implemented in code, MSE might look something like:
def MSE(y_predicted, y):
squared_error = (y_predicted - y) ** 2
sum_squared_error = np.sum(squared_error)
mse = sum_squared_error / y.size
return(mse)
Likelihood Loss
The likelihood function is also relatively simple, and is commonly used in classification problems. The function takes the predicted probability for each input example and multiplies them. And although the output isn’t exactly human interpretable, it’s useful for comparing models.
For example, consider a model that outputs probabilities of [0.4, 0.6, 0.9, 0.1] for the ground truth labels of [0, 1, 1, 0]. The likelihood loss would be computed as (0.6) * (0.6) * (0.9) * (0.9) = 0.2916. Since the model outputs probabilities for TRUE (or 1) only, when the ground truth label is 0 we take (1-p) as the probability. In other words, we multiply the model’s outputted probabilities together for the actual outcomes.
Log Loss (Cross Entropy Loss)
Log Loss is a loss function also used frequently in classification problems, and is one of the most popular measures for Kaggle competitions. It’s just a straightforward modification of the likelihood function with logarithms.
This is actually exactly the same formula as the regular likelihood function, but with logarithms added in. You can see that when the actual class is 1, the second half of the function disappears, and when the actual class is 0, the first half drops. That way, we just end up multiplying the log of the actual predicted probability for the ground truth class.
The cool thing about the log loss loss function is that is has a kick: it penalizes heavily for being very confident and very wrong. Predicting high probabilities for the wrong class makes the function go crazy. The graph below is for when the true label =1, and you can see that it skyrockets as the predicted probability for label = 0 approaches 1.
Loss Functions and Optimizers
Loss functions provide more than just a static representation of how your model is performing–they’re how your algorithms fit data in the first place. Most machine learning algorithms use some sort of loss function in the process of optimization or finding the best parameters (weights) for your data.
For a simple example, consider linear regression. In traditional “least squares” regression, the line of best fit is determined through none other than MSE (hence the least-squares moniker)! For each set of weights that the model tries, the MSE is calculated across all input examples. The model then optimizes the MSE functions––or in other words, makes it the lowest possible––through the use of an optimizer algorithm like Gradient Descent.
Just like there are different flavors of loss functions for unique problems, there is no shortage of different optimizers as well. That’s beyond the scope of this post, but in essence, the loss function and optimizer work in tandem to fit the algorithm to your data in the best way possible.